Keynote Speakers----Dr. Gari Clifford
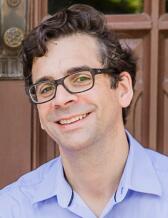
Dr. Clifford is currently the interim Chair of Biomedical Informatics at Emory and an Associate Professor in Biomedical Engineering at Georgia Tech. After training in Mathematical and Theoretical Physics, he received a DPhil in Neural Networks and Biomedical Engineering from the University of Oxford, United Kingdom. He received postdoctoral research training from the Massachusetts Institute of Technology when he later became a Principal Research Scientist, managing the development of the world's largest open access critical care database (MIMIC II). From 2009 to 2014 he served as Associate Professor and Fellow of Kellogg College at the University of Oxford in the Department of Engineering Science, including leading the Intelligent Patient Monitoring Group, and Director of the Centre for Doctoral Training in Healthcare Innovation at the Institute of Biomedical Engineering (IBME). Dr. Clifford has an international reputation in critical care data analysis and the application of signal processing and machine learning to medicine. Dr Clifford helped found the Sleep, Circadian Rhythm and Neuroscience Institute (SCNi) at the University of Oxford, where he is an Honorary Professor.
His research interests include machine learning and signal processing for data fusion, prediction and developing confidence intervals and trust metrics. Application areas in health: critical care, sleep & circadian rhythms, and resource-constrained environments (such as in developing countries). Dr. Clifford leads research on scalable and affordable healthcare: finding ways to use intelligent signal processing, machine learning and physiological modeling to reduce costs, increase accuracy and improve access in healthcare using enormous data streams.
Speech Title: The Problems with (and Solutions for) Digital Cardiology
Abstract: Perhaps the biggest problem with attempts to diagnose patients via automated ECG analysis is the lack of accurate labels on which to train data. PhysioNet (www.physionet.org) has for many years set the gold standard in electrocardiographic (and other) data, leading to the FDA requiring reporting on PhysioNet data. Never-the-less, the last 18 years of PhysioNet/Computing in Cardiology (CinC) Challenges (
www.physionet.org/challenge/) and related work have demonstrated that standard expert labels are far more error prone than would be expected by such an established diagnostic art form. Intra- and Inter-observer disagreements are significant and large, particularly in the more complex (and perhaps important) tasks. With such inconsistencies, it is impossible for a diagnostic system to realistically achieve performance measure above 80-90%, which in turn prevents their use without human oversight; an imperative for large scale analysis. I will discuss solutions to this issue including a voting approach that combines multiple algorithms (and humans) of varying performance levels in an efficient manner to boost labels and classifier performances. It is generally accepted that an ensemble of independent weak classifiers can outperform a single strong classifier, yet little work has been performed on developing mixtures of weak and strong classifiers and measuring and controlling for the correlation between classifiers. I will also discuss this essential element of a label boosting system. Finally, I will discuss the related issue of over-fitting on public (and private) data, which we have observed throughout the literature and even in the PhysioNet/ CinC Challenge test data to some extent. I will therefore propose a generalized framework for testing public algorithms that mitigate this over-training issue and provide realistic estimates of out-of-sample performances.